|
The current status of generative AI in B products At the actual enterprise application level, there are no successful implementation cases or explosive products for B products based on large language models. One reason is the limitations of large language models themselves. Organizing business knowledge into corpus for training large models is a huge project, because a lot of knowledge is tacit and not documented or recorded in writing, but is passed on by word of mouth or face-to-face communication. Organizing this tacit knowledge into training corpus that the
model can understand and digest is a major task. Although the Afghanistan WhatsApp Number quality of the underlying model is good at the level of general transactions and knowledge application, the reality is very skinny when it comes to enterprise-level applications and creating new products. If the enterprise's business knowledge is not effectively transformed into the context required by the model, then using AI for business processing in daily work will not yield the desired results. based on the company's business activities and business pain points. In
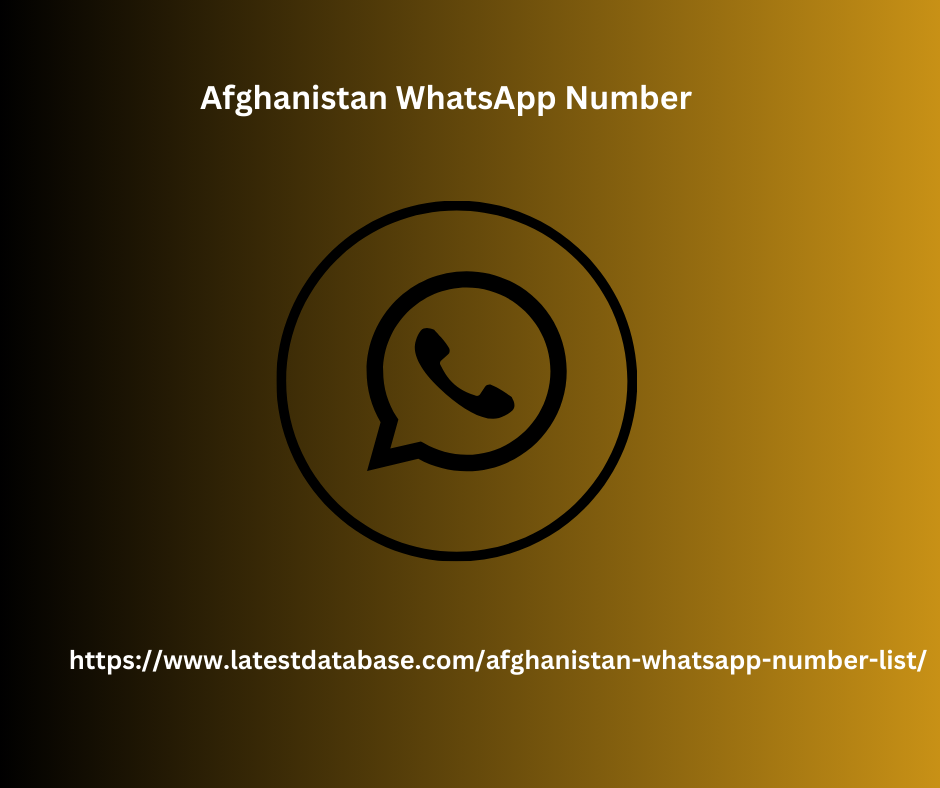
the current product development process, product managers abstract and summarize business logic, and then provide it to R&D personnel for implementation in products. If product managers need to use large language models to design and build products that can be implemented for enterprise users, the first requirement is the reliability of the AI model in terms of business knowledge. My failed product As a product manager, my team and I have tried various application scenarios of AI products on the company’s self-developed business platform. It is a pity that only a few succeed.
|
|